Drug design and development is a complex and costly process that involves identifying and developing new chemical compounds that can be used as drugs to treat diseases. Traditional drug design methods are time-consuming and labor-intensive. Modern drug discovery approaches such as in silico methods have emerged as a way to limit the cost, time, and manpower required in the drug discovery process and hence overcome the drawbacks of traditional approaches.
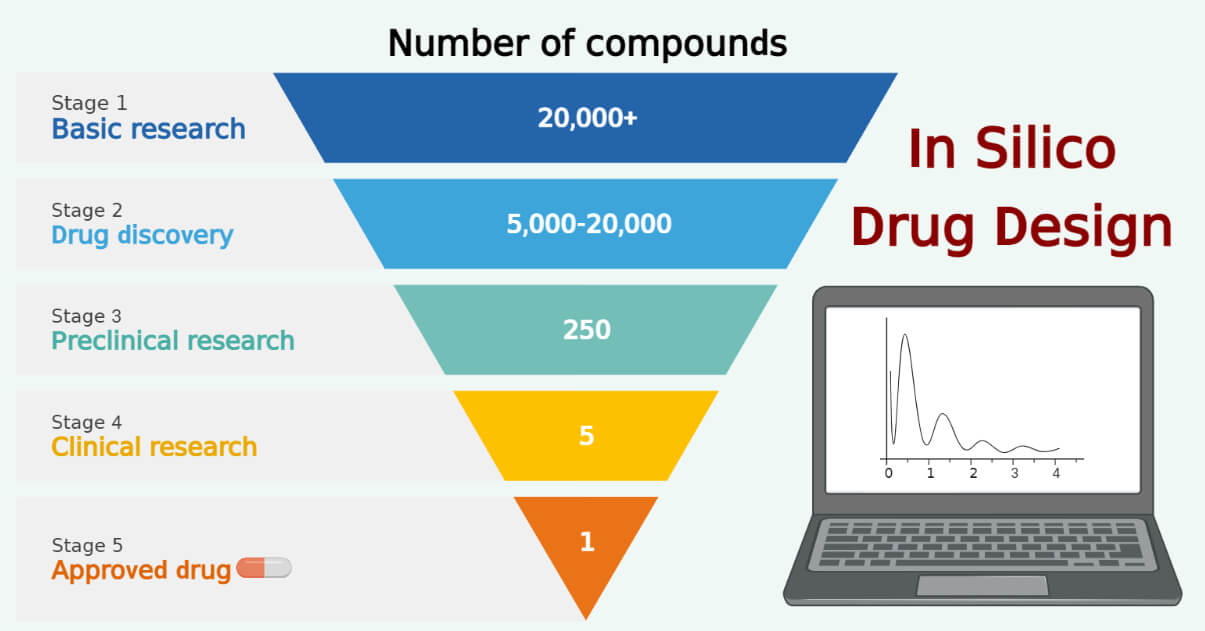
The term “in silico” refers to the use of computer-based experiments to study and analyze biological systems.
In silico drug design, also commonly known as computer-aided drug design (CADD), involves using computational techniques and models to identify drug-like molecules using bioinformatics tools. In silico methods analyze and predict the biological activity of potential drug candidates, and also predict their physicochemical properties.
In Silico Drug Design Methods
Several in silico drug design approaches have been developed. Structure-based drug design (SBDD) and ligand-based drug design (LBDD) are two different methods used.
The choice of a suitable method depends on the availability of target protein structural information. If the three-dimensional structure of the protein is known, SBDD may be the preferred approach as it allows for the design of molecules that fit precisely into the protein’s binding site. If the protein’s structure is not known, LBDD may be the preferred approach as it can be used to design molecules that have similar properties to known ligands that bind to the protein.
Structure-based drug design (SBDD)
SBDD is a method used in drug design to develop new drugs based on the three-dimensional (3D) structure of a target protein that is associated with a specific disease.
The process involves several steps, including the identification of a therapeutic target and active ligands, determination of the 3D structure of the target protein, docking of small molecules into the binding cavity of the target protein, synthesis and optimization of the most promising hits, and evaluation of the biological properties of the selected compounds.
The methods used in SBDD are described below:
- Target determination and structure generation:
- Drug target identification is a crucial step in the drug design process, where a specific biomolecule is targeted for the treatment of a particular disease.
- Once a suitable target is identified, SBDD starts determining and validating the target protein’s 3D structure.
- The 3D structure of the target protein can be determined using both experimental techniques (NMR or X-ray crystallography) and computational techniques (homology modeling and protein folding).
- Binding site prediction:
- The next step is to identify the binding site. A binding site is a specific region on a protein surface where a ligand molecule binds and interacts with the protein to produce a specific biological effect.
- There are several in silico methods for predicting potential binding sites, including docking simulations, molecular dynamics simulations, and protein structure-based algorithms.
- Ligand search:
- Ligand search is the process of identifying potential ligands that can bind to a specific target protein or receptor.
- One of the methods for ligand search is virtual screening (VS), which is a computational approach used to identify potential ligands for drug discovery from large databases of compounds.
- VS involves the screening of large databases of drug-like or lead-like compounds using computational methods to identify molecules that have the potential to bind to a specific target protein with high affinity and specificity.
- Molecular docking:
- Molecular docking is a computational technique used in drug design and screening to predict the interactions between ligands and target proteins or between proteins themselves.
- The goal of molecular docking is to identify potential drug candidates by predicting their ability to form a stable complex with the target biomolecule. It determines the correct binding conformation of ligands and proteins.
- Docking methods are also useful in predicting protein-protein interactions and evaluating the affinity of complexes, thus providing insights into the functional mechanisms and roles of proteins in cells.
- Docking algorithms use scoring functions to assess the binding affinity of the ligands and the target protein which is used to rank the ligands accordingly.
- Fragment-based docking:
- In this approach, molecules are broken down into smaller fragments, and these fragments are screened for binding affinity with the target receptor.
- These fragments generally have lower binding affinity than the entire molecule, but they can provide important information about the key interactions that occur between the molecule and the receptor.
- Scoring function:
- Scoring functions are used to calculate the binding affinity between a ligand and a target protein in molecular docking.
- The accuracy of the scoring function is critical to obtain reliable results from virtual screening experiments.
- Various scoring functions are used and the score generated is crucial for ranking the chemical compounds obtained by virtual screening experiments.
- Molecular dynamics simulations:
- Molecular dynamics (MD) simulation is a computational technique used to study the physical behavior of atoms and molecules in a system, such as protein-ligand complexes.
- MD simulations use mathematical algorithms to calculate the movements of atoms and molecules over time, based on their initial positions and velocities, and the forces acting on them.
- This enables researchers to observe how proteins, drugs, and other molecules interact with each other and their environment under various conditions.
- These simulations can provide insights into the structure and function of proteins, drug-target interactions, and the behavior of molecules.
Ligand-based drug design (LBDD)
LBDD is a method of designing new drugs by using the known structure and functions of a ligand that binds to a target molecule. The ligand serves as a starting point for designing molecules that have similar structural features, which are necessary to interact with the target molecule.
There are several LBDD approaches available, among which pharmacophore modeling, quantitative structure-activity relationship (QSAR), and similarity searches are the more commonly used techniques.
- Pharmacophore modeling
- Pharmacophore modeling involves identifying common structural features of ligands that bind to the target, such as hydrogen bond donors, acceptors, and aromaticity.
- These features are then used to design new molecules that mimic the ligand’s interaction with the target molecule.
- Pharmacophore models are used to screen compound libraries and identify chemicals with similar structural and physicochemical properties that are potential leads for drug discovery.
- To identify pharmacophores, active ligands are computed to generate energetically stable conformations, and then their structures are arranged to identify similar functional groups common to the active ligands. This helps in identifying potential new drug candidates.
- Quantitative Structure-Activity Relationship (QSAR)
- QSAR is a widely used ligand-based method in drug design that generates mathematical models to predict the biological activity of chemical compounds based on their structural and physicochemical properties.
- Molecular descriptors that capture the structural and chemical properties of compounds are calculated, and a mathematical model is constructed that correlates these descriptors with biological activities.
- The model is used to identify new drug candidates and improve the properties of lead compounds.
- It can be used to identify compounds with good inhibitory effects and low toxicity on specific targets.
- A newer version of QSAR methods called 3D-QSAR has been developed to overcome the limitations of traditional QSAR methods.
- Similarity searches
- Similarity searches are computational methods that identify new compounds with similar physicochemical properties to known active compounds.
- The underlying assumption is that molecules with similar properties are more likely to have similar biological activities.
- The process involves comparing the structural and physicochemical properties of known active compounds to a database of compounds.
- Similarity-based methods are not always reliable and may miss compounds with unique chemical features that are important for binding to the target. Therefore, these methods should be used in combination with other computational and experimental techniques for drug discovery.
Applications of in silico drug design
Some of the common applications of in silico methods in drug design and discovery are:
- In silico drug design can be used to screen large databases of compounds and identify potential drug candidates. This approach saves time and resources required for drug discovery.
- In silico drug design can be used to optimize the structure of potential drug candidates to improve their binding affinity, safety, efficacy, and other pharmacological properties.
- In silico methods can be used to predict the binding mode of a drug candidate to a target protein that enables the design of more potent and selective compounds.
- In silico methods can be used to design compounds based on the chemical structure and properties of known ligands that bind to a target protein.
- In silico drug design methods have been used successfully in the design and identification of drug compounds to treat various diseases, including cancer, diabetes, and viral and bacterial infections.
References
- Batool, M., Ahmad, B., & Choi, S. (2019). A Structure-Based Drug Discovery Paradigm. International Journal of Molecular Sciences, 20(11). https://doi.org/10.3390/ijms20112783
- Ekins, S., Mestres, J., & Testa, B. (2007). In silico pharmacology for drug discovery: Methods for virtual ligand screening and profiling. British Journal of Pharmacology, 152(1), 9-20. https://doi.org/10.1038/sj.bjp.0707305
- Ekins, S., Mestres, J., & Testa, B. (2007). In silico pharmacology for drug discovery: Applications to targets and beyond. British Journal of Pharmacology, 152(1), 21-37. https://doi.org/10.1038/sj.bjp.0707306
- Jabalia, N., Kumar, A., Kumar, V., Rani, R. (2021). In Silico Approach in Drug Design and Drug Discovery: An Update. In: Singh, S.K. (eds) Innovations and Implementations of Computer Aided Drug Discovery Strategies in Rational Drug Design. Springer, Singapore. https://doi.org/10.1007/978-981-15-8936-2_10
- Lill, M. A. (2013). In Silico Drug Discovery and Design. doi:10.4155/9781909453012
- Sacan, A., Ekins, S., & Kortagere, S. (2012). Applications and limitations of in silico models in drug discovery. Methods in molecular biology (Clifton, N.J.), 910, 87–124. https://doi.org/10.1007/978-1-61779-965-5_6
- Shaker, B., Ahmad, S., Lee, J., Jung, C., & Na, D. (2021). In silico methods and tools for drug discovery. Computers in biology and medicine, 137, 104851. https://doi.org/10.1016/j.compbiomed.2021.104851
- Singh, S. K. (Ed.). (2021). Innovations and Implementations of Computer Aided Drug Discovery Strategies in Rational Drug Design. doi:10.1007/978-981-15-8936-2
- Yu, W. (2017). Computer-Aided Drug Design Methods. Methods in molecular biology (Clifton, N.J.), 1520, 85. https://doi.org/10.1007/978-1-4939-6634-9_5